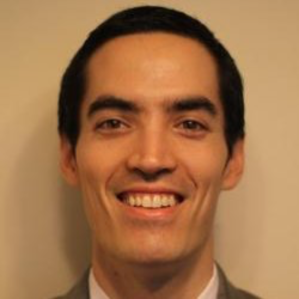
Modeling Customer Memory to Improve Prediction of Customer Purchasing Behavior
Statistics Department; Faculty Advisers: Peter Fader, Shane Jensen
Modeling customer purchase behavior is very important to retailers. Armed with a better understanding of future expected purchase behavior, retailers can estimate important statistics like customer lifetime value, allowing for more informed marketing decisions. However, three of the most well known and well used models for customer purchase behavior do not take into account the fact that customer preferences may change, instead assuming that customer preferences remain constant – that they are ‘stationary’. We propose a statistically principled way of allowing for non-stationarity in customer preferences by accounting for what we call ‘customer memory’. Customer memory is the notion that more recent behavior is a more important indicator of the future than past behavior, all else equal. This will allow us to not only make better predictions, it will also allow us to see whether and how much customer preferences change together – that is, whether there are so-called customer purchasing ‘contagion effects’.
Publications
McCarthy, Daniel and Peter Fader (2017), “Valuing Non-Contractual Firms Using Common Customer Metrics,” Working Paper, https://papers.ssrn.com/sol3/papers.cfm?abstract_id=2923466
Presentations
“V(CLV): Examining Variance in Models of Customer Behavior”, Marketing Strategy Meets Wall Street Conference, Singapore (January 2015)
“V(CLV): Examining Variance in Models of Customer Behavior”, Marketing Science Conference, Baltimore (June 2015)