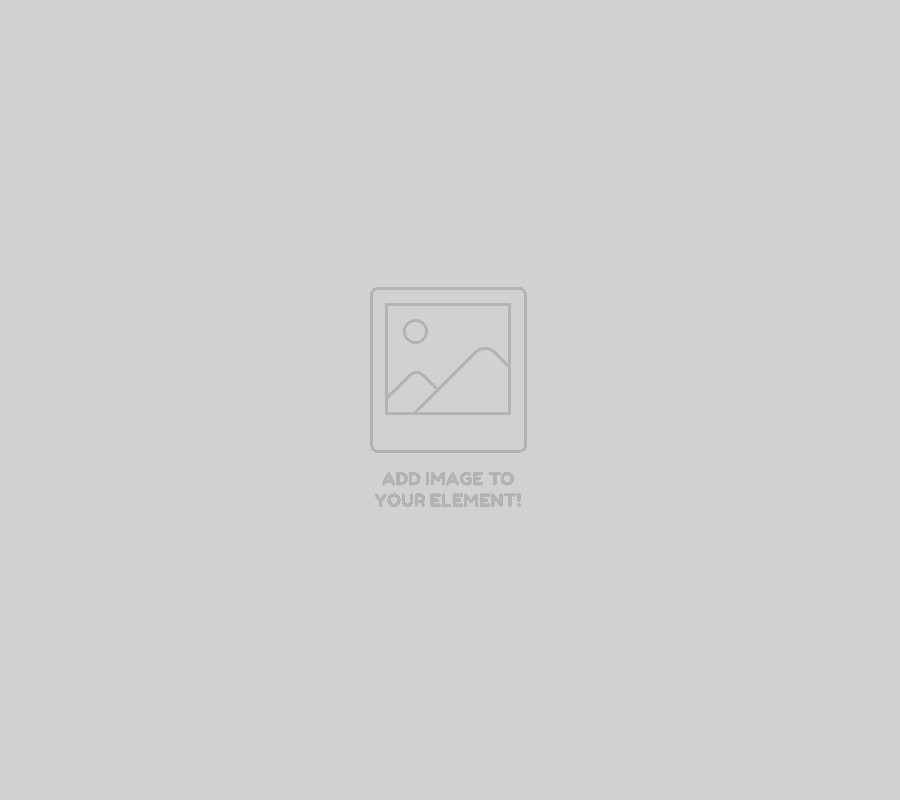
A Novel Approach to Multi-Source Attribution
Operations and Information Management Department; Faculty Adviser: Raghuram Iyengar
Consumers often make decisions under the influence of multiple sources (e.g., promotions, ads and friends) and it’s important for managers to identify the contribution of each source in the decision process. Despite the obvious managerial implications of the multi-source attribution problem in consumers’ decision-making process, there is limited past research primarily due to the lack of a suitable methodological framework. In this paper, we propose a general reduced-form regression model that can be easily applied to various kinds of multi-source attribution contexts. The proposed model is an extension of the popular proportional hazards model, in the sense that it allows the cause of an event to be multiple sources. Our model has several advantages as compared to past modeling efforts in multi-source attribution. First, the model does not speculate on the contribution of each channel apriori, but lets the data automatically determine the contribution of individual channel based on its characteristics. Second, it is easy to use and interpret as compared to structural-form models for attribution, and more rigorous as compared to previous rule-based attribution methods. Third, it provides unbiased estimates even if only part of the sources contributes to the conversion of consumers. Fourth, the model can easily estimate the probabilistic contribution of each source. We demonstrate the effectiveness of our model using two real-world datasets.
Publications
Peng, Jing and Raghuram Iyengar (2018), “A Novel Approach to Multi-Source Attribution,” Working Paper.
Presentations
Marketing Science Conference, 2018
Symposium on Statistical Challenges in Electronic Commerce Research, 2018
China Summer Workshop on Information Management, 2015
Workshop on Information Technologies and Systems, 2014
Symposium on Statistical Challenges in Electronic Commerce Research, 2014