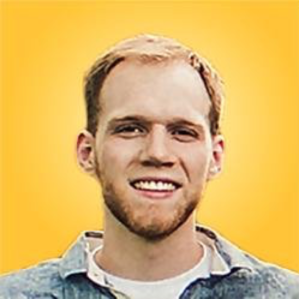
An Empirical Meta-Analysis of Online E-commerce Experimentation
Operations, Information, & Decisions Department; Faculty Adviser: Kartik Hosanagar
In this project, we attempt to provide a rigorous, empirical analysis of e-commerce A/B testing practices. To accomplish this, we perform a meta-analysis on nearly 2000 A/B tests conducted by more than 200 e-commerce companies across seven industries over the course of more than three years. While there is much interest in the field of digital experimentation generally, little is known about the types of experiments that firms actually run on their websites, which of these are most effective, and how these results may interact with important factors. Our goal with this project is to answer these questions.